At the Perceptual Neuroscience Lab, our research focuses on understanding the dynamic interplay between top-down and bottom-up processes using behavioral experiments, functional magnetic resonance imaging (fMRI), and computational modeling. See some relevant readings:
- Urgen, BM, Boyaci, H. (2021). Unmet expectations delay sensory processes. Vision Research, 181, 1-9, https://doi.org/10.1016/j.visres.2020.12.004
- Urgen, BM, Boyaci, H (2021). A recurrent cortical model can parsimoniously explain the effect of expectations on sensory processes. bioRxiv, https://doi.org/10.1101/2021.02.05.429913
The Influence of Physical and Semantic Regularities on Perception, Learning, and Decision-Making
Physical and semantic regularities are deeply embedded in our everyday experiences, such as the consistent relationship between light and shadows or the contextual association of objects like books in a library. These regularities play a critical role in how we perceive and interpret our surroundings, adapt through perceptual learning, and make decisions. Our research aims to uncover the mechanisms through which these environmental patterns shape perception, guide learning, and influence decision-making using psychophysics and computational approaches.
The Role of Prior Knowledge in Perceptual Decision-Making
We investigate how prior knowledge and expectations shape perceptual decision-making processes. Through psychophysics and computational modeling, we aim to resolve inconsistencies reported in the literature (e.g., the neural effects of expectations) by systematically comparing different methodological approaches, including variations in stimuli, tasks, and experimental paradigms. Our objective is to identify the underlying factors driving these discrepancies and to enhance our understanding of these complex mechanisms.
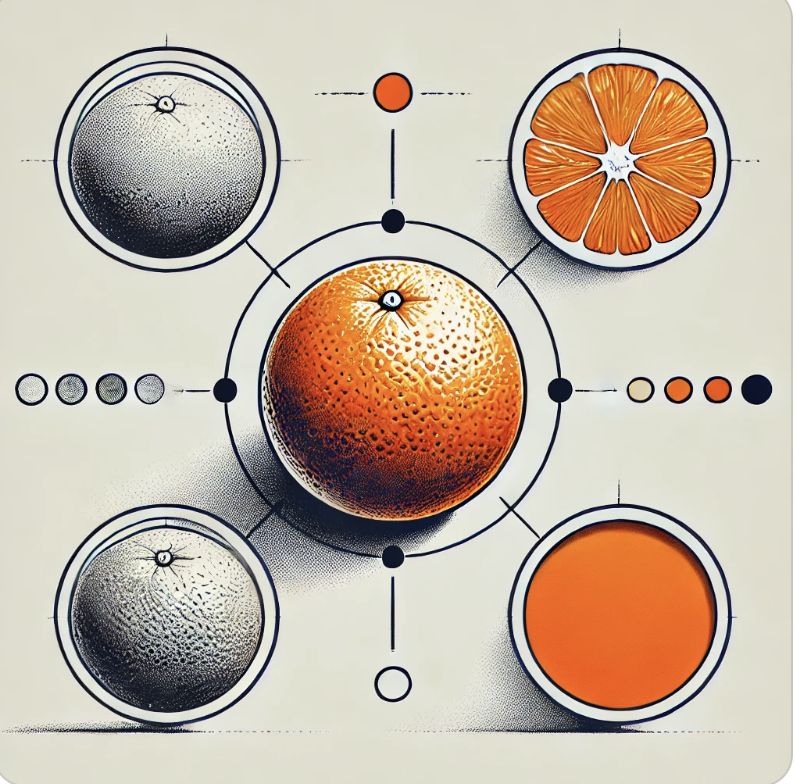
Effects of Attention and Expectations on Object Perception
We explore how high-level cognitive processes shape mechanisms underlying object recognition. Specifically, we investigate how these processes influence early sensory representations, facilitating the identification and categorization of objects in our environment. By integrating psychophysics and fMRI, this work aims to enhance our understanding of how the brain efficiently processes complex visual information to support object recognition and interpretation.
Predictive Processing Across Psychiatric Disorders and Healthy Aging
The predictive processing framework provides a theoretical basis for understanding how the brain integrates sensory inputs and predictions to process information. While this framework has been applied to explain neural mechanisms underlying psychiatric disorders, it also offers valuable insights into how these mechanisms evolve with healthy aging.
Our research focuses on exploring predictive processing components—such as the interaction between sensory inputs and predictions, the determination of prediction precision, and their integration—and investigating how these processes differ across psychiatric disorders and age-related cognitive changes. To address these questions, we use psychophysics, computational modeling, and fMRI to test and refine these theoretical models.